Reimagining Mesoamerican and Colonial Historical Archaeology with Artificial Intelligence
Artificial Intelligence (AI) is reshaping how we explore the past, offering innovative tools to decipher vast historical archives, map forgotten geographies, and uncover hidden narratives. In historical archaeology, particularly the study of colonial Mesoamerica, AI techniques are revolutionizing the way we analyse and understand complex datasets from centuries-old documents.
The Challenge of Historical Big Data
The 16th-century Relaciones Geográficas de Nueva España, commissioned by Philip II of Spain, exemplifies historical big data. This collection includes around 2.8 million words spread across 168 textual reports and 78 maps, encompassing detailed descriptions of geography, governance, trade, and Indigenous traditions. While rich in information, these documents pose challenges for researchers due to their sheer volume, linguistic diversity, and historical context. Traditional methods of archival study, including the palaeography and analysis at large scale of such vast, complex datasets, usually take researchers a life time to explore and accomplish. This is where AI is entering historical archaeology as a transformative force.

Natural Language Processing (NLP): Teaching AI to Read History
The "Digging into Early Colonial Mexico" project leveraged a suite of AI methods from approaches in natural language processing (NLP), computer vision, corpus linguistics, and geographic information systems (GIS), to automatically identify information, extract, analyse and map data from the Relaciones. By combining methods from these fields, researchers can extract meaningful insights from historical texts and images at scales previously unattainable. The cornerstone of this effort is Geographical Text Analysis (GTA), a method and software that integrates identification of concepts with NLP, computational linguistics, and spatial data to identify geographic references in texts and connect them with historical contexts [read more about it here].
Using GTA, researchers can explore questions such as: “What diseases were mentioned in specific regions, and how were they treated?” or “What economic activities were prevalent in colonial towns, and how did these vary geographically?” These questions allow for a multidimensional analysis of historical phenomena, revealing patterns and relationships that would have been impossible to discern through manual methods alone.
At the heart of GTA is natural language processing (NLP), a subfield of AI that focuses on teaching computers to understand and interpret human language. NLP uses machine learning algorithms to analyse text, identify patterns, and extract meaningful information. For historical archaeology, NLP is enabling us to analyse massive collections of documents, identifying keywords, concepts, and relationships without the need for manual annotation of every record.
As part of the Relaciones project, NLP techniques were employed to annotate historical texts with specific categories such as plants, diseases, architecture, people’s names, animals, and geographic features, among many others. This annotation process involves training the AI on a curated sample of texts, where human experts define and label key terms and categories. Once trained, the AI can process thousands of unseen documents, automatically identifying mentions of topics of interest.

Geographical Text Analysis software created by the Digging into Early Colonial Mexico Project. The software allows the automated query of information through semantic concepts, keywords, or text strings and their connection to possible geographies in thousands of pages.
For instance, in studying colonial epidemics, the project’s NLP system identified over 300 unique terms related to diseases. By linking these terms with geographic data, we have mapped the spread and perception of diseases like smallpox across colonial Mexico.This integration of textual and spatial analysis is transforming our understanding of how Indigenous and Spanish communities experienced and responded to the 16th century epidemics.

Geographic Information Systems project showing the mapping of the mention of diseases in the 16th century corpus of the Geographic Reports of New Spain.
Computer Vision: Analysing Visual Histories
While NLP focuses on text, computer vision is transforming the analysis of visual records in historical archives. Computer vision is a Machine Learning field that enables machines to interpret and analyse visual data, such as images, videos, and handwritten text. This technology can be pivotal in unlocking the wealth of information contained in maps, codices, and hybrid pictorial documents from colonial archives.
In the Relaciones, many maps blend Indigenous pictorial traditions with European cartographic styles, creating unique visual records that combine symbolic and geographic elements. Through an approach called Visual Natural Language Processing (Visual NLP) [read more about the approach here], we are teaching AI systems to recognize and interpret these complex images. For instance, the AI can identify toponyms (place names), architectural features, and symbolic motifs, linking these elements to textual and geographic data.
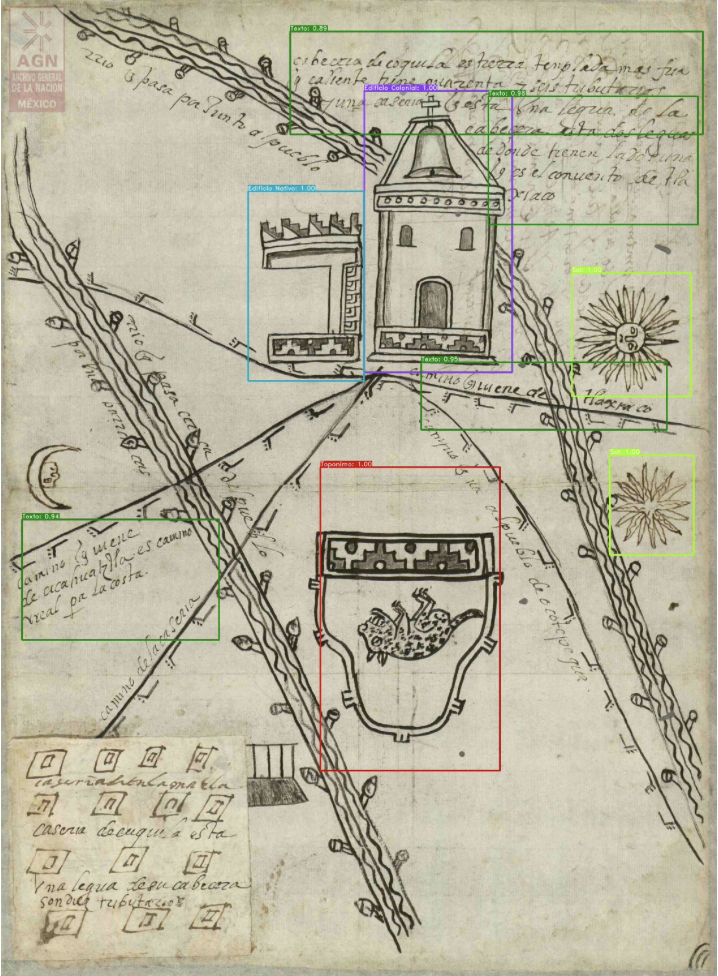
Example of machine learning model for object recognition in the colonial map of Coquila. Plano de Coquila, Tlaxiaco, Oaxaca (1599). AGN, Tierras, vol. 3556, exp.6, f. 175
This integration of visual and textual analysis allows for a deeper exploration of colonial documents. Researchers can study how Indigenous and Spanish mapping traditions merged, how mythological and geographic narratives intertwined, and how these visual records functioned as tools for governance and identity formation.
Handwritten Text Recognition (HTR): Unlocking Manuscripts
One of the greatest challenges in historical research is the abundance of documents in archives, the majority of which remain untranscribed and therefore, unexplored. To carry out the transcription of documents researchers must spend years specialising in palaeography, deciphering obscure penmanship and the vicissitudes of historical language. Machine learning has also the potential of transforming this. Handwritten Text Recognition (HTR) is a specialized AI technique designed to convert handwritten text into machine-readable formats. Unlike optical character recognition (OCR), which works well for printed texts, HTR is trained to recognize the variability and complexity of handwritten scripts.
In the Unlocking the Colonial Archive project, HTR has been a game-changer. Using machine learning, the project has trained AI models to read 16th-century Spanish American documents with different types of calligraphies, as well as Indigenous scripts and mixed-language documents. These models are tailored to specific handwriting styles, such as Italic or Procesal, ensuring high accuracy despite the diversity of scripts.
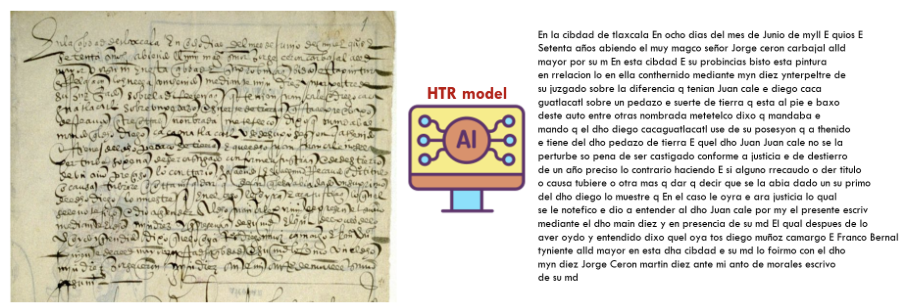
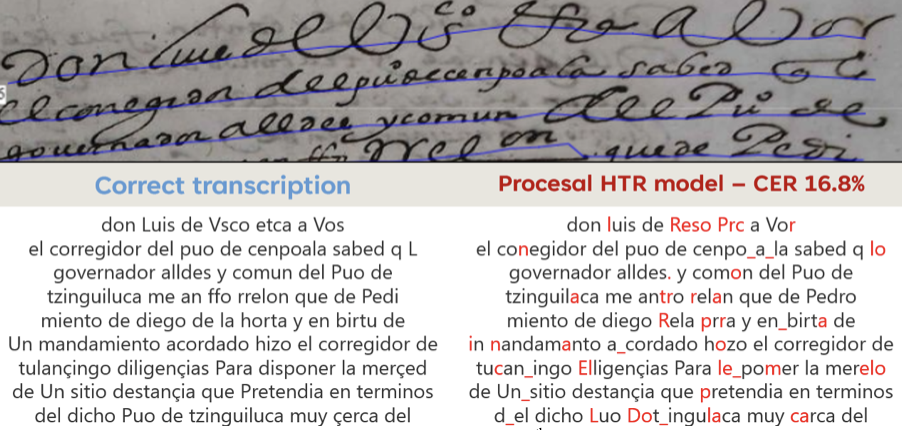
For example, HTR models can enable the automatic transcription of historical legal documents, land titles, and personal letters, making these resources accessible for analysis. In one instance, researchers used HTR to extract references to land disputes in colonial legal records, linking these with geographic data to map territorial changes over time. This not only accelerates the pace of research but also democratizes access to archival materials, allowing more scholars and communities to engage with historical sources.
Empowering Communities and Democratizing Knowledge
AI tools in historical archaeology are not just about academic research—they also offer opportunities for community engagement and knowledge sharing. Many Indigenous communities continue to use colonial documents, such as maps and codices, as part of their cultural and legal practices. Recognizing this, our research groups are collaborating with Indigenous communities to integrate knowledge into digital archives and the creation of open online platforms for community use.
For instance, community members can contribute with insights on the meanings of Indigenous place names, the symbolism in pictorial documents, and the oral histories associated with colonial records. At the same time, communities will have authority control over the information they input in the platforms and can decide to share it, or not with the wider world. These collaborations ensure that AI-driven analyses respect and incorporate CARE principles, Indigenous perspectives, creating a more inclusive and holistic understanding of history.
A Future Shaped by AI and Collaboration
As AI continues to advance, its applications in historical archaeology will only grow more sophisticated. By combining NLP, computer vision, HTR, and GIS, projects like the examples presented here, are creating new possibilities for exploring the past. These tools allow researchers to analyse massive datasets, uncover hidden patterns, and connect fragmented historical records across time and space. Furthermore, with the introduction of Large Language Models and the boom of Generative AI, other possibilities are opening up to work with large volumes of historical texts.
However, the true potential of AI lies in its ability to foster collaboration—between historians, archaeologists, computer scientists, and the communities whose histories these archives represent. Together, these diverse voices are reshaping how we remember, study, and engage with the past. This is the case of a recent project called ‘The New Spain Fleets: Delving into three centuries of socioeconomic history through Artificial Intelligence’ where a collaboration between many disciplines are taking place.
AI can be not just a tool for uncovering history but a bridge that connects us to the stories, cultures, and peoples who shape our world. As we continue to reimagine historical archaeology with AI, we are not only advancing scholarship but also ensuring that history remains a living, dynamic dialogue for generations to come.