April 2025. New Technological Milestones in Historical Mapping
We moved away from our previous method of generating historical maps using image generation models due to their unreliable and hard-to-control results. The earlier approach, which combined state-of-the-art maps with historical points, faced challenges with source verification, inconsistent formats, and synchronization of historical names. As a result, we decided to retain only the historical points as a foundation for a new method.
After assessing resources and data collection costs, we developed a new approach to building historical maps based on how historians work—by identifying places mentioned as part of a state in a given year and using geography to infer borders.
Our method gathers historical place data, extracts yearly subsets with place names and associated states, and then applies a nearest-neighbour clustering algorithm to generate maps. This approach offers more control and historical accuracy than image-based generation.
Data gathering
Our data gathering process involves creating a list of historical places with basic timelines using ChatGPT, then verifying and enriching this data with local DeepSeek and Phi-4 models via Wikipedia. This iterative process improves precision, and once the dataset is solid, we’ll incorporate written sources for further validation and correction. We also plan to develop a system to verify historical facts against our database.
To build accurate maps, our system first corrects state name inconsistencies and groups synonymous names. Standard clustering algorithms don’t work for our needs, so we adapted a Nearest Neighbour approach with two point types and optimized distance calculations. For modern maps (post-1800), we apply a fast iterative expansion algorithm to fill in gaps, as seen in examples like Mesopotamia in -2000 or the British Empire in North America in 1900.
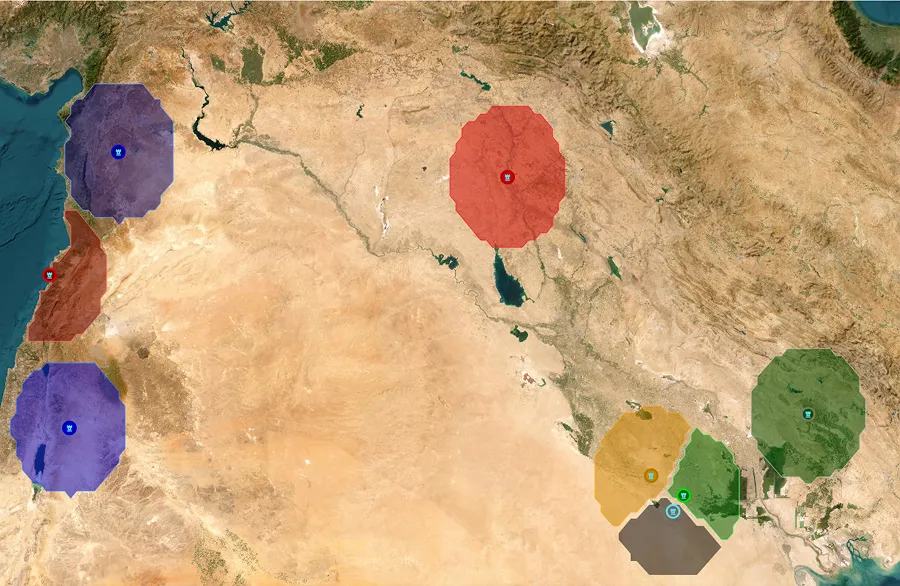
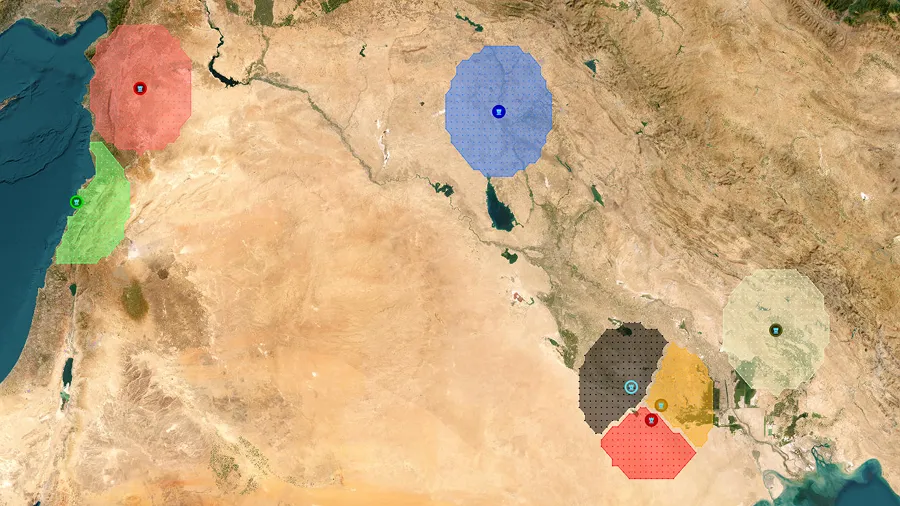


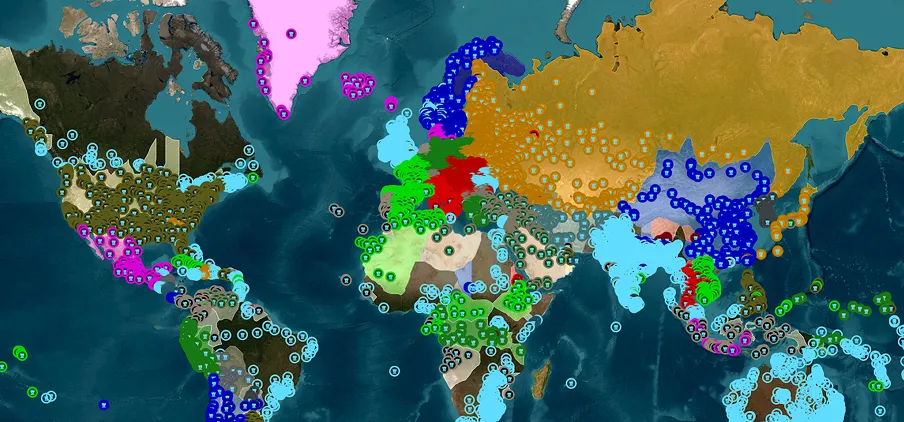
Our approach faces several challenges, such as inconsistent labels (e.g., Prague being part of Bohemia, the Kingdom of Bohemia, or the Holy Roman Empire), which we resolve by standardizing to the highest political authority using verification steps. To handle Earth’s scale, we optimized our custom clustering and map expansion algorithms for speed. Issues like colonial borders, sparsely inhabited regions, nomadic states, and chaotic historical periods (e.g., WWI, USSR collapse) require tailored solutions, including iterative expansion and variable time intervals for data gathering.
Conclusion
We plan to release the first map preview in a few weeks and update it every two weeks as we refine the algorithm and data. This method can also be used to map wars, religions, plagues, population density, languages, and archaeological cultures.